Who We Help
Case Studies
About Us
Insights
About
Pricing
Feature [CMS]
Feature Details [CMS]
Integration
Team
Blog [CMS]
Blog Details [CMS]
Contact v1
Contact v2
FAQ
Privacy
Terms
404
Who We Help
Case Studies
About Us
Insights
About
Pricing
Feature [CMS]
Feature Details [CMS]
Integration
Team
Blog [CMS]
Blog Details [CMS]
Contact v1
Contact v2
FAQ
Privacy
Terms
404
Preventing Denials with Revenue Cycle Analytics
Preventing Denials with Revenue Cycle Analytics
Preventing Denials with Revenue Cycle Analytics
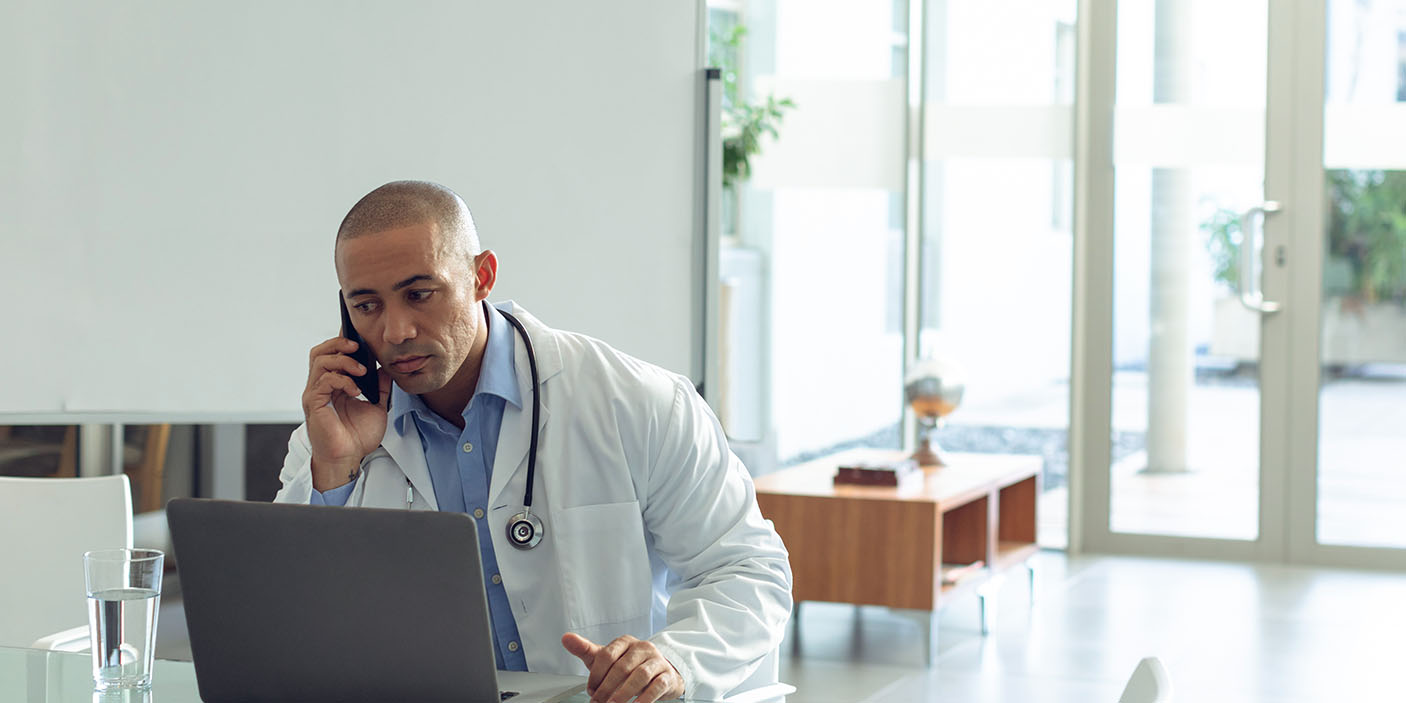
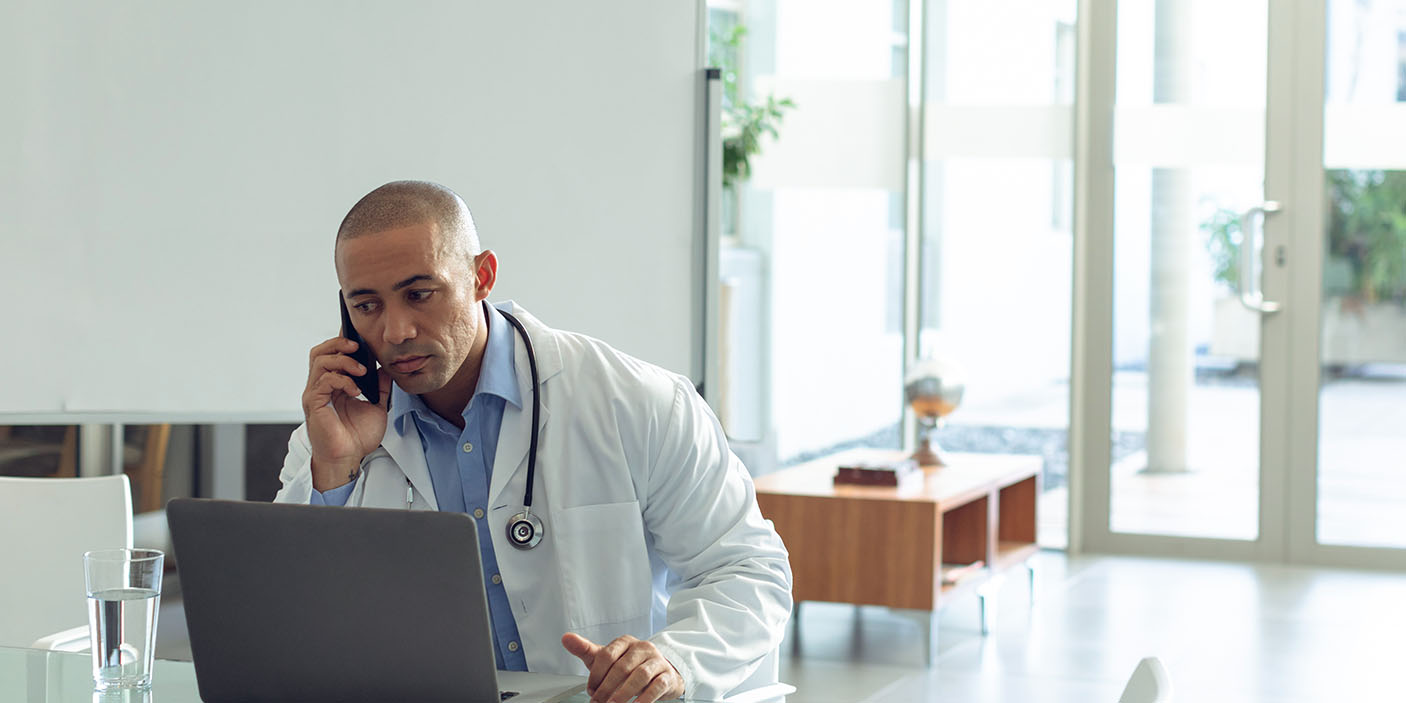
Executive Summary:
Healthcare organizations face increasing denial rates, impacting financial health. This article explores using data analytics to identify recurring denial patterns and improve reimbursement outcomes. By leveraging internal data and predictive revenue cycle analytics, providers can proactively address issues before they result in denials. This approach optimizes processes, reduces denials, and enhances revenue cycle management, ultimately improving patient outcomes and financial stability.
Introduction
Healthcare organizations in the US face a lot of denials. This is because of a continuous battle between insurers and policy makers - like a game of cat and mouse.
However, even the most volatile reimbursement relationship can be better managed. The trick is following specific rules to achieve reimbursement. Everyone must play this game - providers (in-network and out-of-network) and payers too.
Our goal is to identify how we can use data to understand a payer's response to a claim. We can use this data to modify our internal processes and stop future denials.
Let's start with the good news. Healthcare organizations are data powerhouses.
Every patient interaction generates a colossal amount of data. Each data point attaches to a patient's record. This data accumulates over time and increases with every patient visit.
Each of these visits generates meaningful order data, which in turn converts into claim data. This claim data transmits to the payer, which returns even more data back. In the form of a denial (or approval), along with more data.
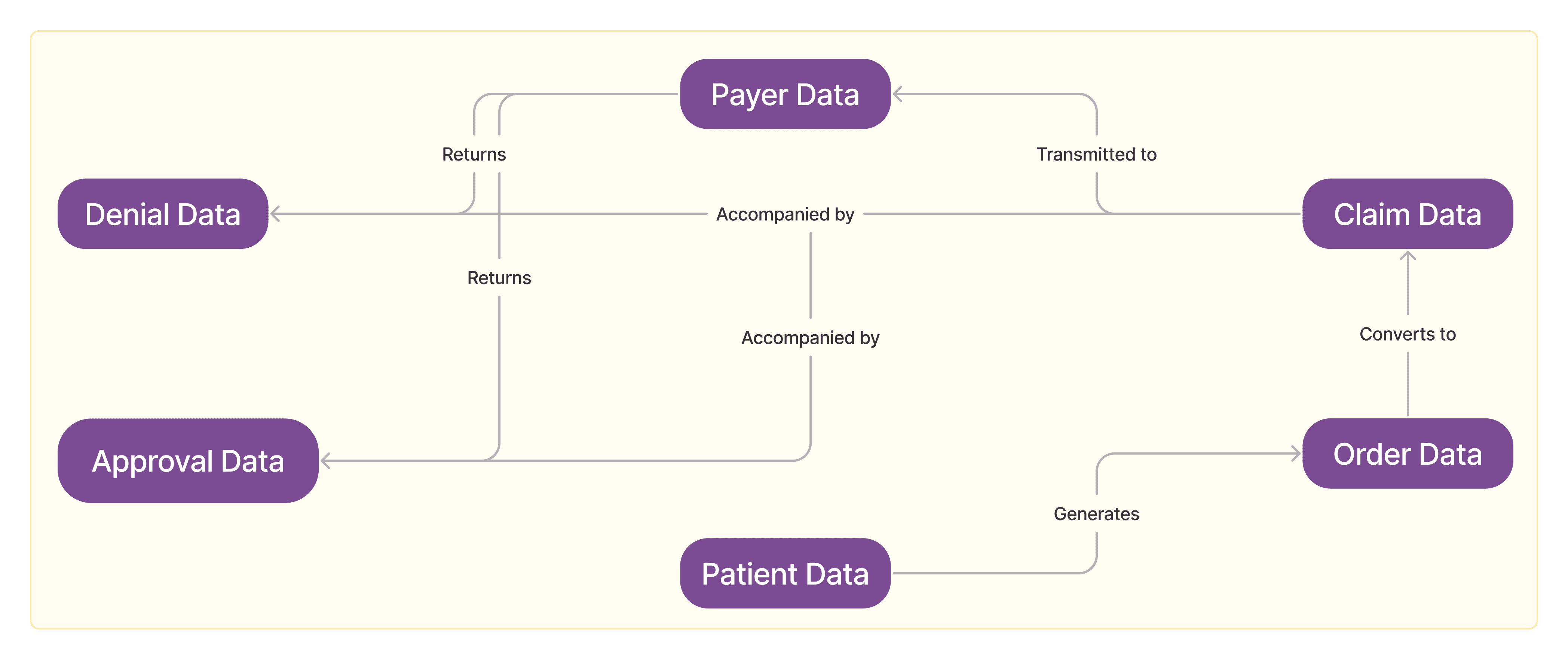
You get the picture - but this is still just a snapshot. This simple illustration is to show you how a single patient generates many data records. Now multiply this by 10x for real life scenarios, and then by your patient population.
But don’t miss the most important point. This data is instrumental. These are the building blocks we can use to help avoid denials.
The ability to access the data, the quality of that data and the access to expertise pose a massive challenge. Without the right approach rendering them useless in driving meaningful impact for healthcare organizations.
In this piece, we'll explore how your own internal data can prevent claim denials. We'll delve into how to probe your data, ask pertinent questions, and understand how your operational workflows impact outcomes.
Let's examine how to use order, patient, insurance, adjudication, and payer data to enhance reimbursement outcomes.
Decoding Denials: Data as Your Detective
The average denial rate has increased to 12% in the first half of 2022. Compare this to 10% in 2020 and 9% in 2016. (Source: Change Healthcare).
And that’s only the average - there are providers that are at the 30% denial rate and over.
Thankfully we now can use data to help us identify recurring denial patterns. By recognizing these patterns, providers can delve into the root causes of denials and develop strategies to prevent future occurrences.
Denials vary; each one presents a unique circumstance. But within this diversity patterns emerge, revealing important insights.
For example, in 2022 the most common reasons for denials were:
Lack of medical necessity (27%)
Incorrect coding (24%)
Out-of-network services (18%)
Preauthorization requirements (14%)
The average time it took to resolve a denial was 45 days.
So how could data give us daily guidance on what’s going on with our denials?
Let's take a look at this real-time revenue cycle KPI dashboard on Gistia RevenueIQ. It’s telling us that the average payment rate is trending in the right direction - a 3.6% increase. Cash trends are improving; however, charge volume is down (yet still above the target).
But if you look closely, the denial rate is higher than expected. It shot over our 20% target. Why?
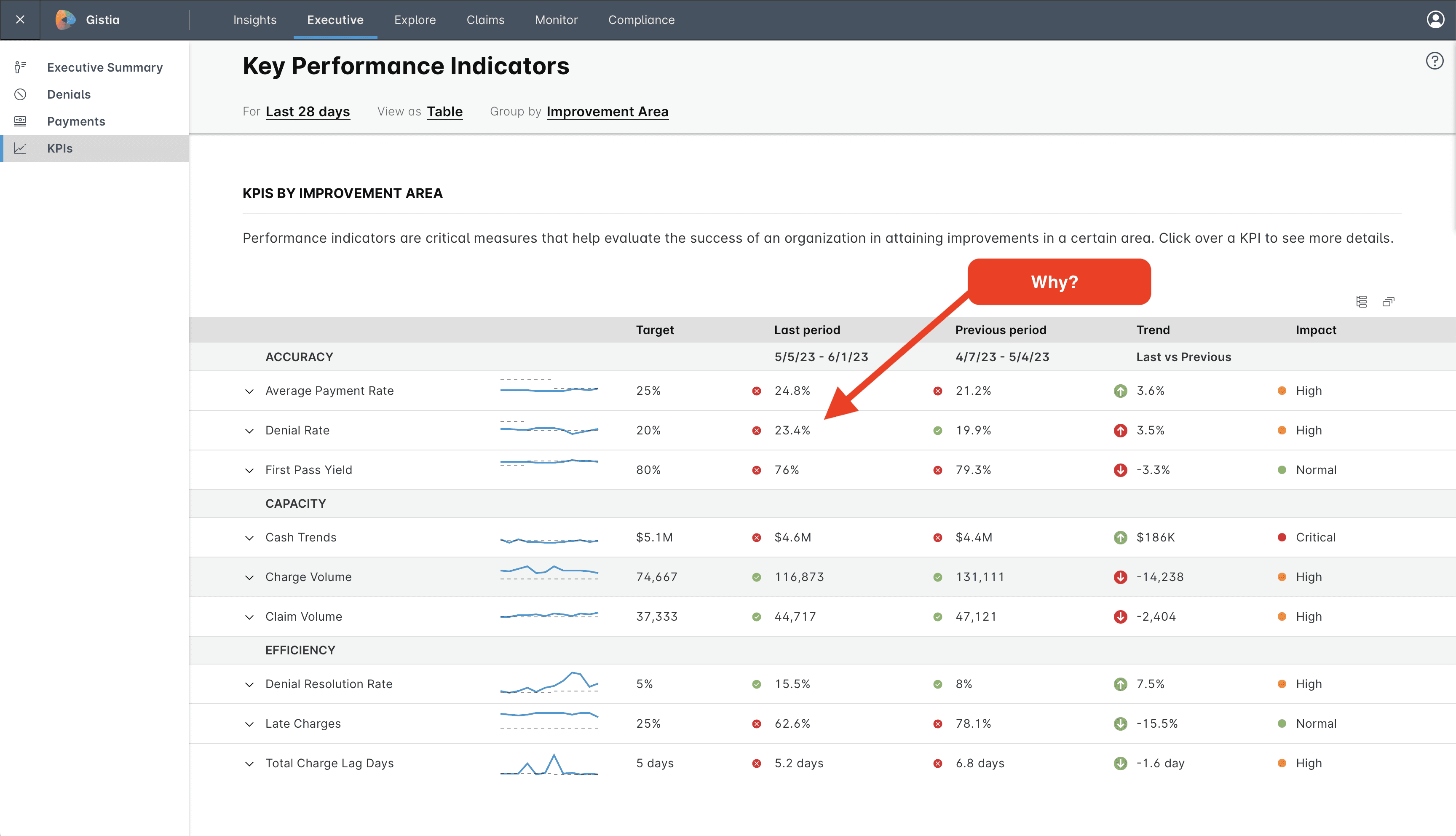
Armed with this information, we can now dig deeper into answering the key questions.
Predictive Revenue Cycle Analytics: A Glimpse into the Future
Predictive revenue cycle analytics can revolutionize the identification of claims at risk of denial. This foresight allows providers to spot and correct potential issues before submitting their claims.
This insight extends beyond understanding the past—it's about predicting and shaping the future. Unveiling root causes means proactive measures can be taken to prevent recurring denials. This may involve changes in operational processes, staff training, or patient communication.
Providers repeatedly denied a certain service may be showing a pattern. The pattern could include repeated denial codes from a specific insurer. Additionally, the combination of CPT codes and ICD codes may be the same for all claims from a particular patient demographic.
The root cause could be insufficient documentation or missing prior authorization. For example, perhaps a non-invasive prenatal test is performed, but the patient's sex is male. There is now a problem with the ICD code and the patient's sex.
By leveraging data, the provider can identify where in their processes the data inputs are driving these denials and take action. Doing so will reduce denials, improve revenue, increase patient satisfaction, and aid in operational efficiency.
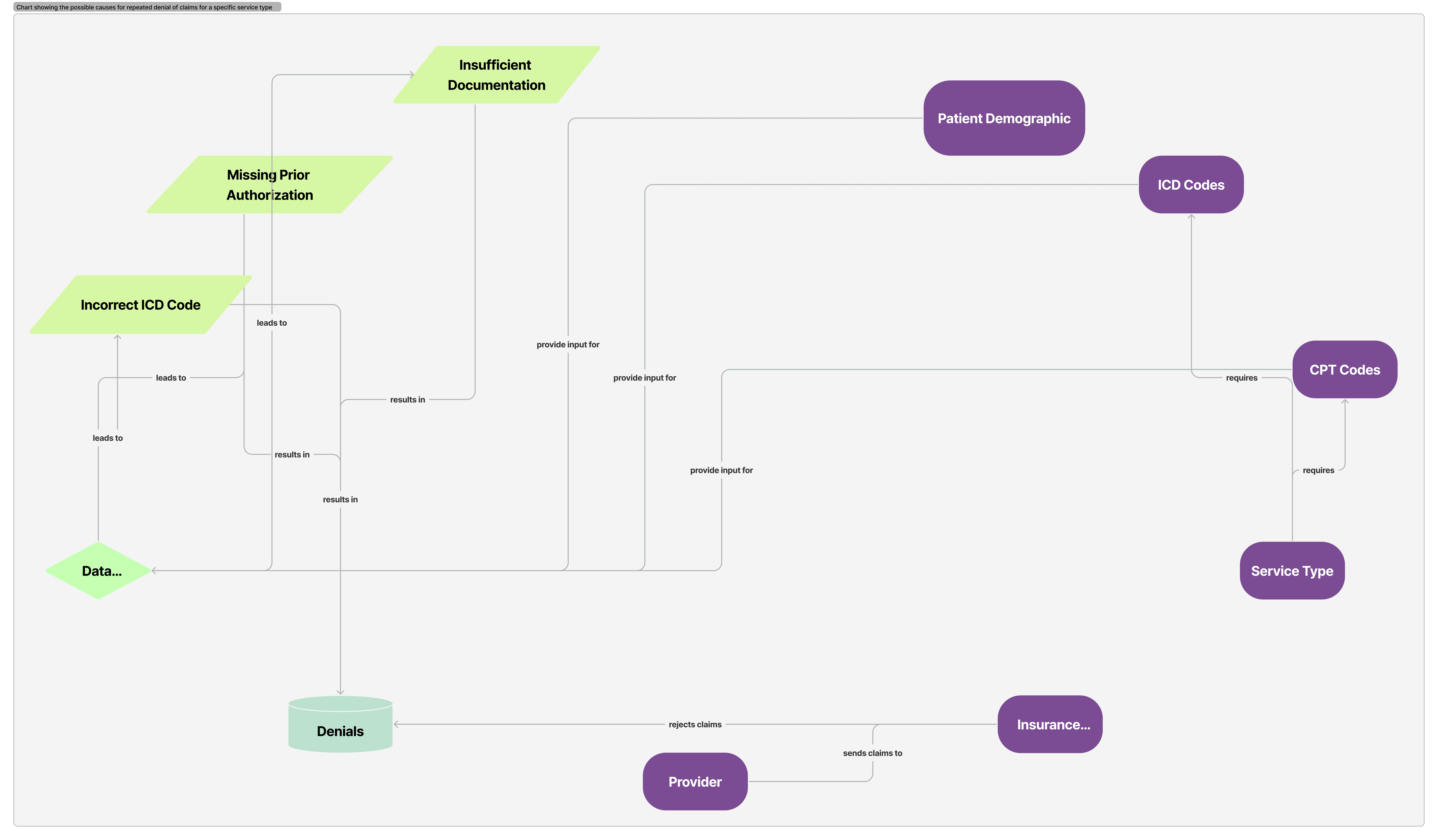
Predictive revenue cycle analytics isn't just traditional data analysis though—it projects us into the future. Picture a typical day in a healthcare provider's office. Amid thousands of claims, potential denials lurk.
Predictive RCM analytics are especially effective at identifying at-risk claims early. This gives ample opportunity to address any issues before they lead to denials.
Moreover, predictive revenue cycle analytics facilitates the shift from a reactive approach to a proactive one. We're no longer hastily solving problems after they occur. Instead, we're predicting and preventing them, enhancing cash flow and patient satisfaction.
Predictive revenue cycle analytics empowers us to stay a step ahead in the challenging game of claims submission and reimbursement. It's about predicting the future, making informed decisions, and skillfully navigating the complex terrain of claim submissions.
Use Predictive Revenue Cycle Analytics as Your Guide
A fascinating element of revenue cycle management lies in the untapped potential of metadata—the information about the data. This is where revenue cycle analytics really shines, providing invaluable insights to augment your operations. Utilizing revenue cycle analytics (specifically predictive analytics), systems can capture the often-overlooked metadata generated from order and claim workflows. This robust approach can provide precise guidance on where adjustments are necessary, right down to individual data elements.
Imagine the possibility of having a dynamic feedback loop. This loop connects the last step of a process back to its inception, creating an unbroken cycle of continuous improvement. Here, we find predictive revenue cycle analytics playing a pivotal role.
Focus on the quality of data throughout the revenue cycle. This provides important insights into potential issues.
Recognizing the importance of data quality in driving outcomes is fundamental. Stay mindful of the context in which you're assessing data quality. Ensure the data you use is accurate, comprehensive, and pertinent. This increases its effectiveness in driving operations towards a positive outcome.
Simply put, the use of predictive revenue cycle analytics is about understanding what triggers claim denials, in-depth. This knowledge becomes an essential tool in preemptively addressing these triggers, thus preventing future denials. It goes beyond a superficial understanding, diving deep into the nuances of data to reveal patterns and trends.
The transformation of raw data into actionable insights is the cornerstone of a proactive approach, fostered by revenue cycle analytics. This strategic method continually optimizes our processes, reduces denials, and refines revenue cycle management. The analytics enable us to identify problem areas, understand them, and take preventive measures to curb their recurrence.
By using predictive revenue cycle analytics, we're not just reacting to the present; we're anticipating the future. Revenue cycle analytics is important for predicting future trends and potential problems. This allows organizations to refine processes, reduce denials, and improve the efficiency of revenue cycle management.
Envisioning a Brighter Future: Better Patient Outcomes with Revenue Cycle Analytics
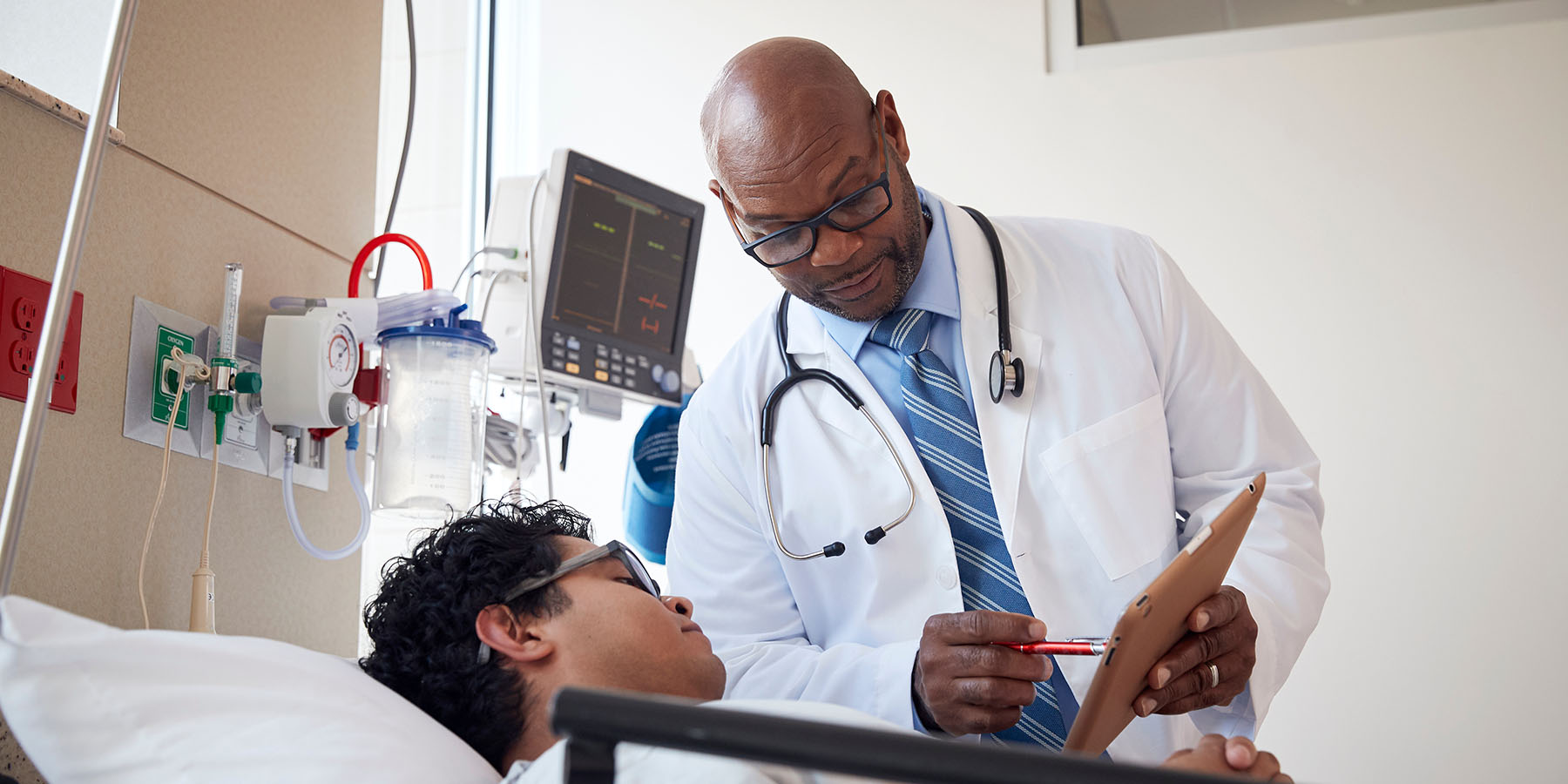
Data harnessed correctly will improve revenue cycle management. New technologies are key. A robust analytics strategy such as revenue cycle analytics can maximize effectiveness and reduce claim denials.
However, the benefits of integrating these tools extend beyond mere cost reduction and efficiency enhancement. More significantly, it opens up avenues to increased revenue generation and fosters financial stability.
Importantly, it's crucial not to overlook another profound benefit of employing revenue cycle analytics – the potential for enhanced patient care. Ensuring the provision of services is seamless, we can effectively eliminate needless delays. This ensures patients receive their much-needed care in a timely manner. By improving processes, we are, in effect, improving patients' experiences and outcomes.
In the infancy of AI and machine learning, the time for action is now. Healthcare providers should seriously consider investing in innovative technologies, especially ones such as revenue cycle analytics. These aren't just tools; they're catalysts for transformation.
They have the power to improve workflows, reduce mistakes, and bring about meaningful change. Technology will fundamentally change how we view and manage our revenue cycle.
It's not just about keeping pace with the ever-evolving world of healthcare technology. It's about actively shaping the future through the thoughtful adoption of innovation. By integrating revenue cycle analytics into our toolkit, we can not only react to changes but anticipate them. This proactive approach ensures that we stay ahead, driving improvements in our processes, patient outcomes, and bottom lines.
The future of healthcare is data-driven, and revenue cycle analytics stands at the forefront. We can use the power of healthcare providers to create a financially secure future. This will also lead to a healthcare ecosystem that is more focused on the needs of patients.
A Quick Win: Gistia RevenueIQ
Gistia RevenueIQ is a low-risk, quick-win solution. It uses revenue cycle analytics to improve revenue cycle management.
Gistia RevenueIQ helps you prepare for future trends, prevent claim denials, and make workflows faster. This will benefit your organization and, most importantly, the patients you serve. It will create a brighter and more sustainable future.
Frequently Asked Questions
How can data help prevent claim denials in healthcare organizations?
Data can help us identify patterns in denials. This allows us to uncover their root causes. We can then develop strategies to prevent them from happening again. By recognizing these patterns, providers can make necessary adjustments to their internal processes.
What is predictive revenue cycle analytics?
Predictive revenue cycle analytics is a method that uses historical data. By applying advanced algorithms it can identify denied claims.
Providers can anticipate potential issues before submitting their claims. This foresight allows them to take a proactive approach to revenue cycle management. Correcting issues beforehand prevents problems down the line.
How can metadata be useful in revenue cycle analytics?
Metadata, or information about the data, can provide invaluable insights to augment operations. Predictive revenue cycle analytics can help improve data-driven decision-making. It does this by focusing on the quality of data in the context of the entire revenue cycle. This provides crucial insights into potential problem areas.
What are the benefits of using revenue cycle analytics in healthcare organizations?
Benefits include the ability to identify problem areas, understand them, and take preventive measures to curb their recurrence. This strategic and ongoing method optimizes processes, reduces denials, refines revenue cycle management, and ultimately leads to improved patient outcomes.
How can revenue cycle analytics improve patient care?
Processes improve - eliminating needless delays and ensuring patients receive care quickly. This contributes to improved patients' experiences and outcomes.
Preventing Denials with Revenue Cycle Analytics
Preventing Denials with Revenue Cycle Analytics
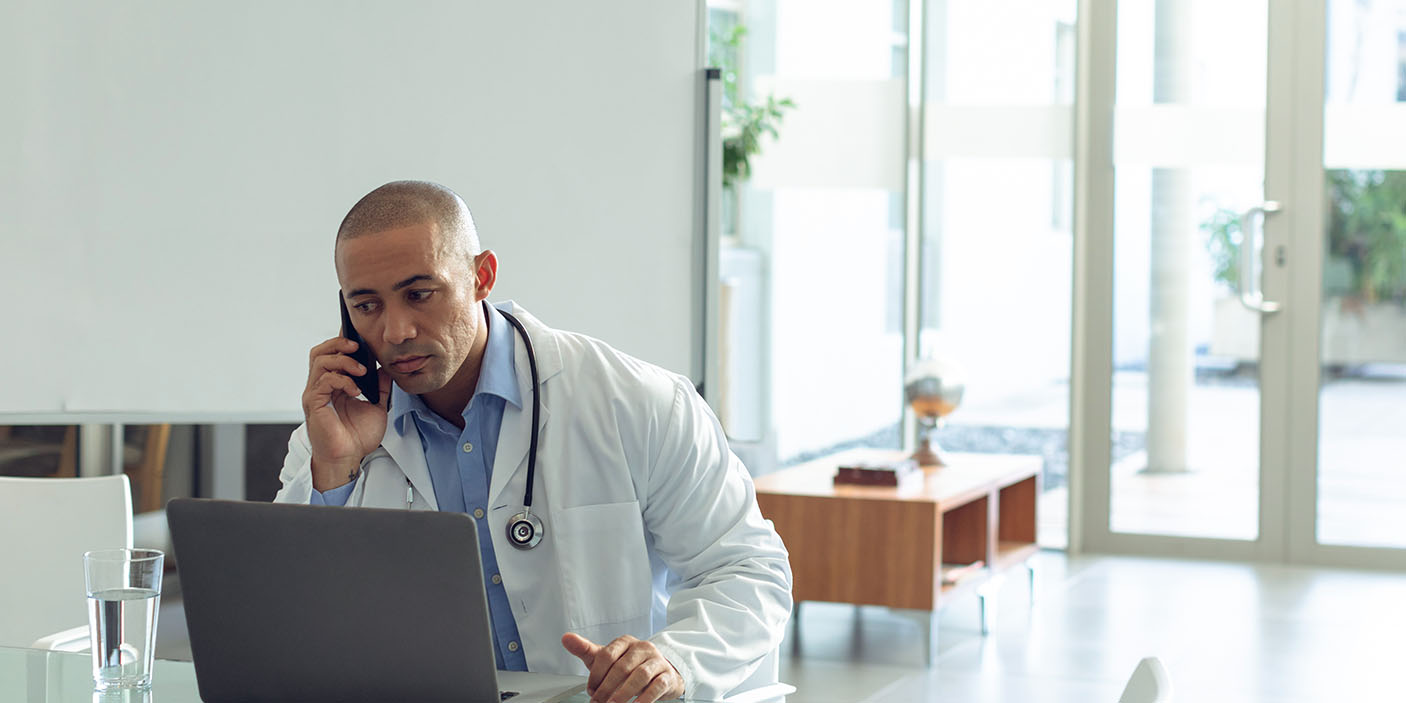
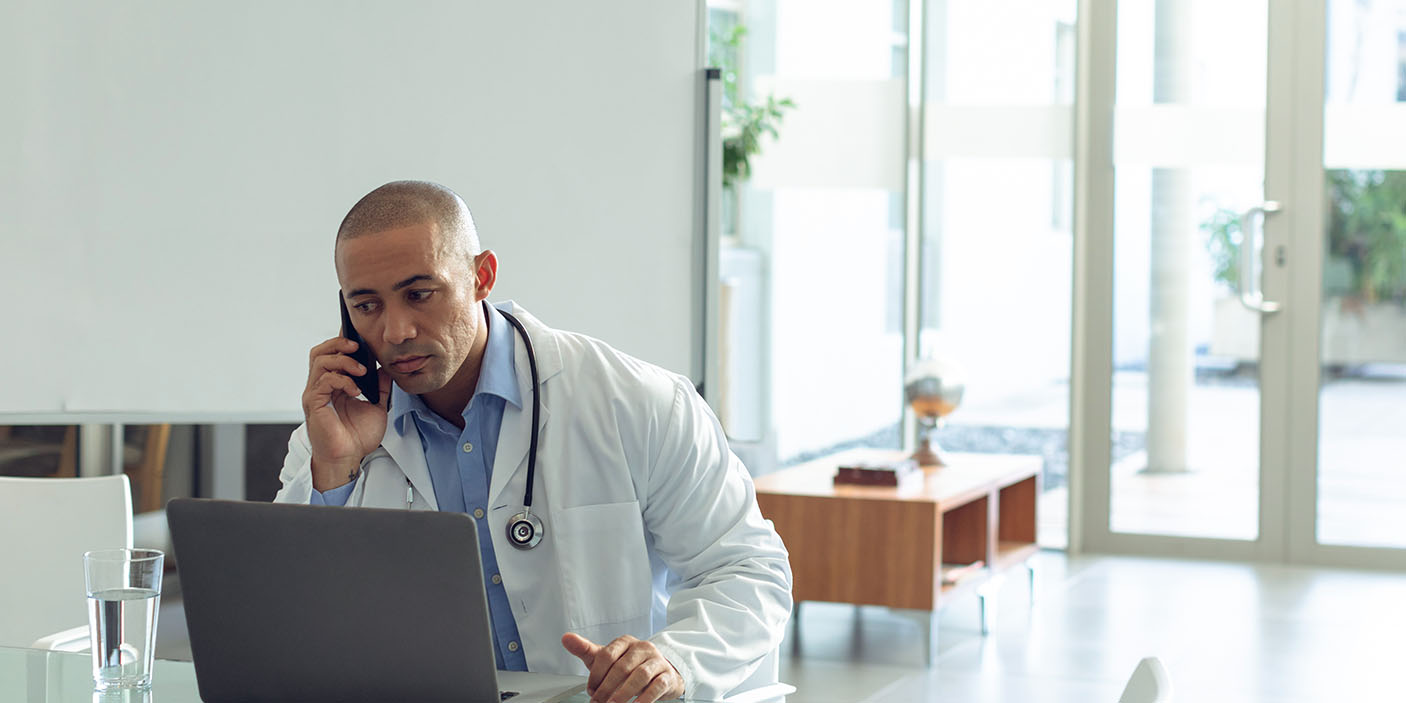
Executive Summary:
Healthcare organizations face increasing denial rates, impacting financial health. This article explores using data analytics to identify recurring denial patterns and improve reimbursement outcomes. By leveraging internal data and predictive revenue cycle analytics, providers can proactively address issues before they result in denials. This approach optimizes processes, reduces denials, and enhances revenue cycle management, ultimately improving patient outcomes and financial stability.
Introduction
Healthcare organizations in the US face a lot of denials. This is because of a continuous battle between insurers and policy makers - like a game of cat and mouse.
However, even the most volatile reimbursement relationship can be better managed. The trick is following specific rules to achieve reimbursement. Everyone must play this game - providers (in-network and out-of-network) and payers too.
Our goal is to identify how we can use data to understand a payer's response to a claim. We can use this data to modify our internal processes and stop future denials.
Let's start with the good news. Healthcare organizations are data powerhouses.
Every patient interaction generates a colossal amount of data. Each data point attaches to a patient's record. This data accumulates over time and increases with every patient visit.
Each of these visits generates meaningful order data, which in turn converts into claim data. This claim data transmits to the payer, which returns even more data back. In the form of a denial (or approval), along with more data.
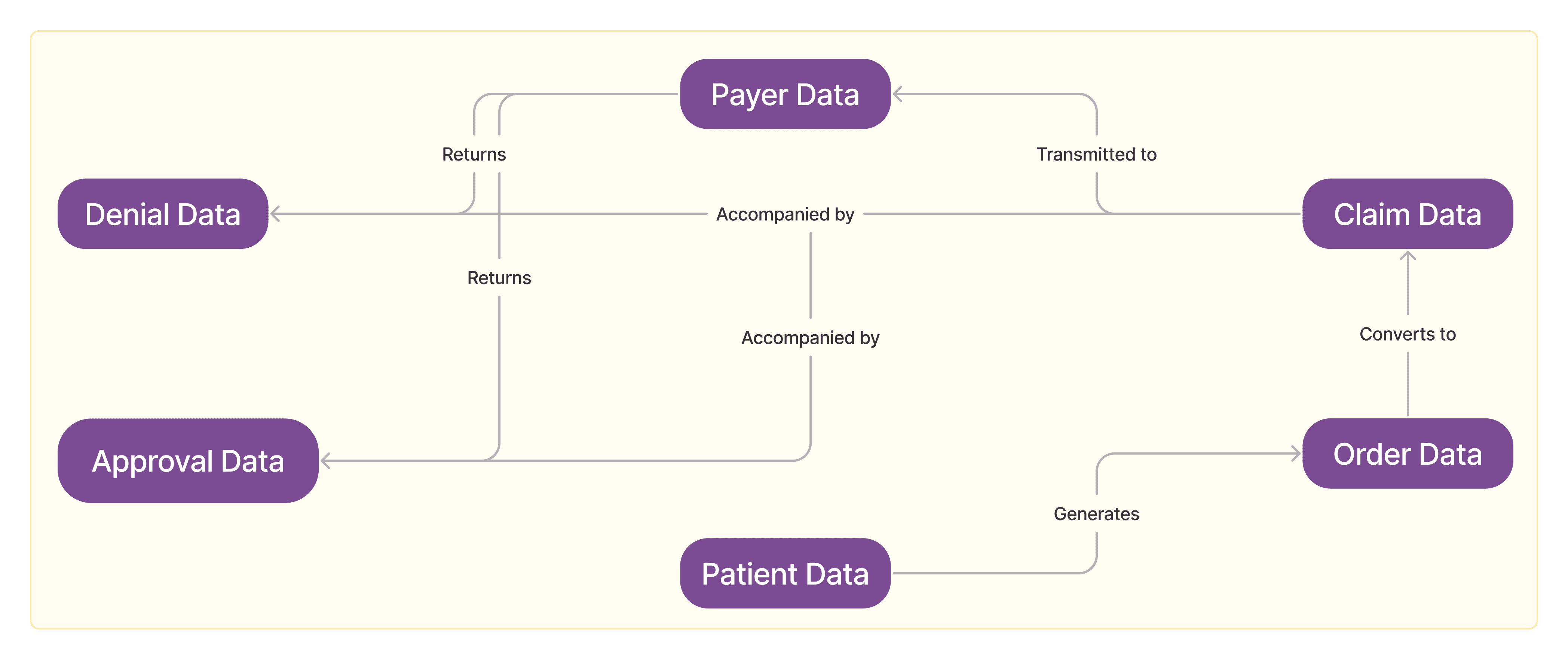
You get the picture - but this is still just a snapshot. This simple illustration is to show you how a single patient generates many data records. Now multiply this by 10x for real life scenarios, and then by your patient population.
But don’t miss the most important point. This data is instrumental. These are the building blocks we can use to help avoid denials.
The ability to access the data, the quality of that data and the access to expertise pose a massive challenge. Without the right approach rendering them useless in driving meaningful impact for healthcare organizations.
In this piece, we'll explore how your own internal data can prevent claim denials. We'll delve into how to probe your data, ask pertinent questions, and understand how your operational workflows impact outcomes.
Let's examine how to use order, patient, insurance, adjudication, and payer data to enhance reimbursement outcomes.
Decoding Denials: Data as Your Detective
The average denial rate has increased to 12% in the first half of 2022. Compare this to 10% in 2020 and 9% in 2016. (Source: Change Healthcare).
And that’s only the average - there are providers that are at the 30% denial rate and over.
Thankfully we now can use data to help us identify recurring denial patterns. By recognizing these patterns, providers can delve into the root causes of denials and develop strategies to prevent future occurrences.
Denials vary; each one presents a unique circumstance. But within this diversity patterns emerge, revealing important insights.
For example, in 2022 the most common reasons for denials were:
Lack of medical necessity (27%)
Incorrect coding (24%)
Out-of-network services (18%)
Preauthorization requirements (14%)
The average time it took to resolve a denial was 45 days.
So how could data give us daily guidance on what’s going on with our denials?
Let's take a look at this real-time revenue cycle KPI dashboard on Gistia RevenueIQ. It’s telling us that the average payment rate is trending in the right direction - a 3.6% increase. Cash trends are improving; however, charge volume is down (yet still above the target).
But if you look closely, the denial rate is higher than expected. It shot over our 20% target. Why?
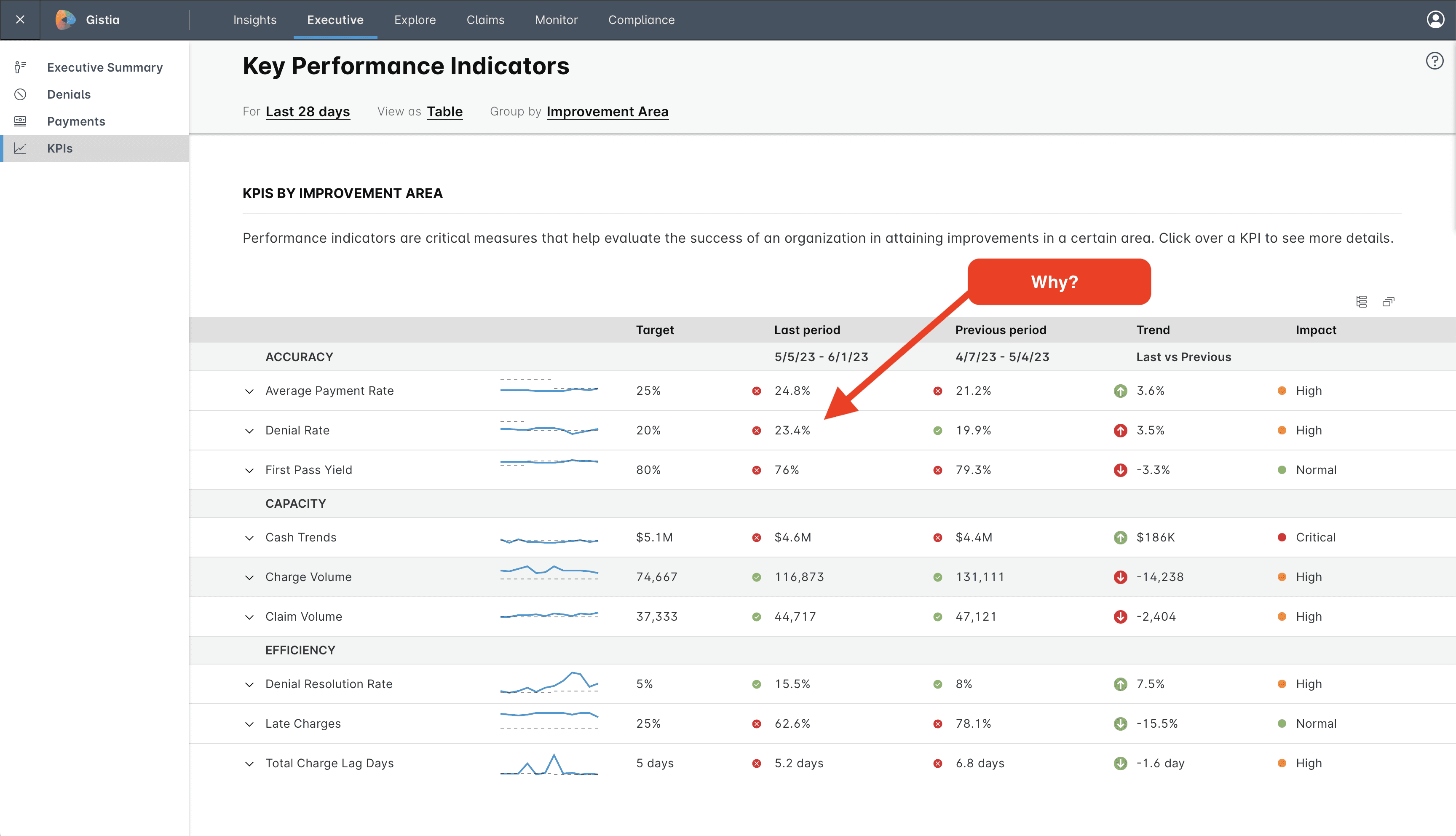
Armed with this information, we can now dig deeper into answering the key questions.
Predictive Revenue Cycle Analytics: A Glimpse into the Future
Predictive revenue cycle analytics can revolutionize the identification of claims at risk of denial. This foresight allows providers to spot and correct potential issues before submitting their claims.
This insight extends beyond understanding the past—it's about predicting and shaping the future. Unveiling root causes means proactive measures can be taken to prevent recurring denials. This may involve changes in operational processes, staff training, or patient communication.
Providers repeatedly denied a certain service may be showing a pattern. The pattern could include repeated denial codes from a specific insurer. Additionally, the combination of CPT codes and ICD codes may be the same for all claims from a particular patient demographic.
The root cause could be insufficient documentation or missing prior authorization. For example, perhaps a non-invasive prenatal test is performed, but the patient's sex is male. There is now a problem with the ICD code and the patient's sex.
By leveraging data, the provider can identify where in their processes the data inputs are driving these denials and take action. Doing so will reduce denials, improve revenue, increase patient satisfaction, and aid in operational efficiency.
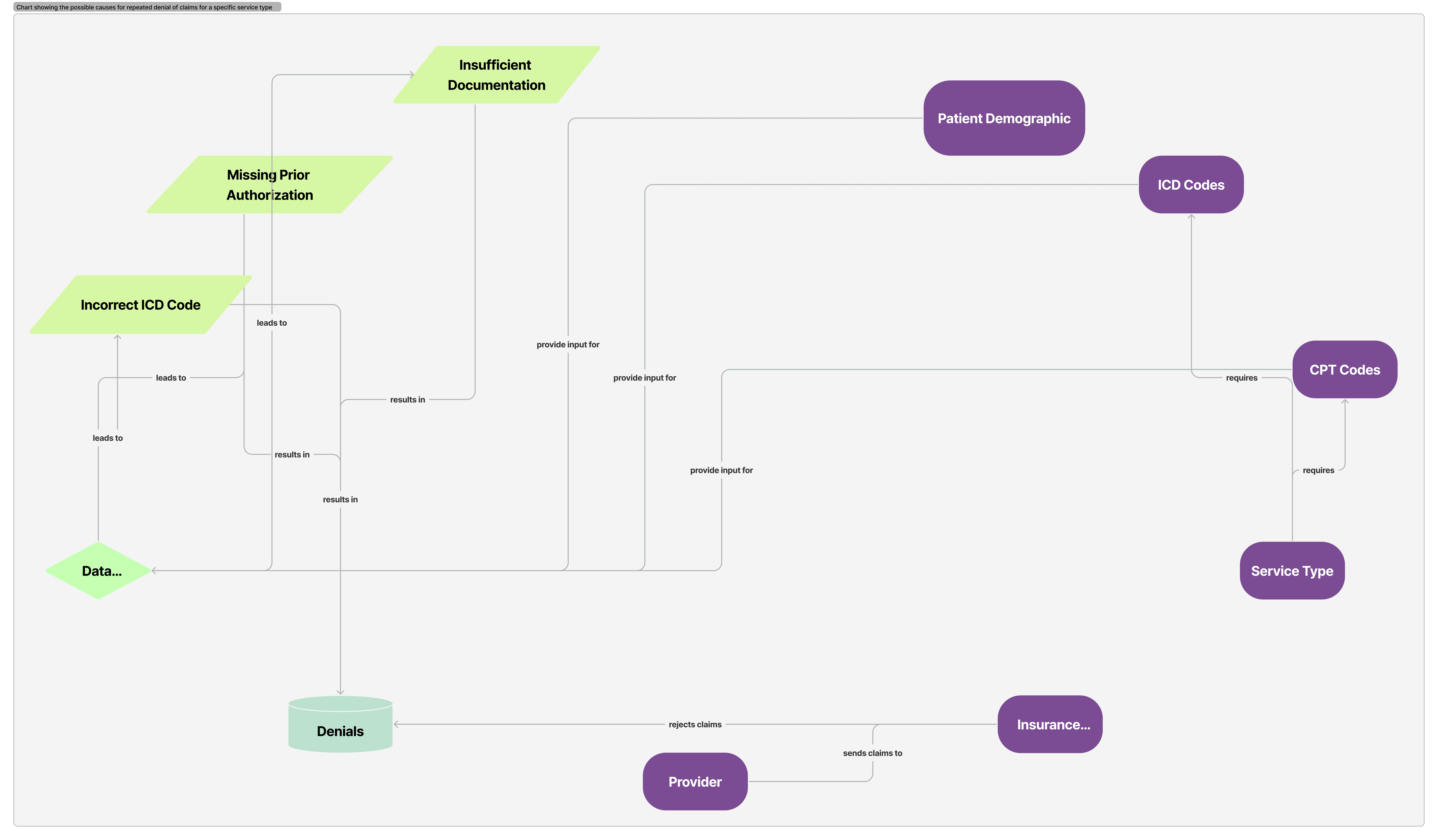
Predictive revenue cycle analytics isn't just traditional data analysis though—it projects us into the future. Picture a typical day in a healthcare provider's office. Amid thousands of claims, potential denials lurk.
Predictive RCM analytics are especially effective at identifying at-risk claims early. This gives ample opportunity to address any issues before they lead to denials.
Moreover, predictive revenue cycle analytics facilitates the shift from a reactive approach to a proactive one. We're no longer hastily solving problems after they occur. Instead, we're predicting and preventing them, enhancing cash flow and patient satisfaction.
Predictive revenue cycle analytics empowers us to stay a step ahead in the challenging game of claims submission and reimbursement. It's about predicting the future, making informed decisions, and skillfully navigating the complex terrain of claim submissions.
Use Predictive Revenue Cycle Analytics as Your Guide
A fascinating element of revenue cycle management lies in the untapped potential of metadata—the information about the data. This is where revenue cycle analytics really shines, providing invaluable insights to augment your operations. Utilizing revenue cycle analytics (specifically predictive analytics), systems can capture the often-overlooked metadata generated from order and claim workflows. This robust approach can provide precise guidance on where adjustments are necessary, right down to individual data elements.
Imagine the possibility of having a dynamic feedback loop. This loop connects the last step of a process back to its inception, creating an unbroken cycle of continuous improvement. Here, we find predictive revenue cycle analytics playing a pivotal role.
Focus on the quality of data throughout the revenue cycle. This provides important insights into potential issues.
Recognizing the importance of data quality in driving outcomes is fundamental. Stay mindful of the context in which you're assessing data quality. Ensure the data you use is accurate, comprehensive, and pertinent. This increases its effectiveness in driving operations towards a positive outcome.
Simply put, the use of predictive revenue cycle analytics is about understanding what triggers claim denials, in-depth. This knowledge becomes an essential tool in preemptively addressing these triggers, thus preventing future denials. It goes beyond a superficial understanding, diving deep into the nuances of data to reveal patterns and trends.
The transformation of raw data into actionable insights is the cornerstone of a proactive approach, fostered by revenue cycle analytics. This strategic method continually optimizes our processes, reduces denials, and refines revenue cycle management. The analytics enable us to identify problem areas, understand them, and take preventive measures to curb their recurrence.
By using predictive revenue cycle analytics, we're not just reacting to the present; we're anticipating the future. Revenue cycle analytics is important for predicting future trends and potential problems. This allows organizations to refine processes, reduce denials, and improve the efficiency of revenue cycle management.
Envisioning a Brighter Future: Better Patient Outcomes with Revenue Cycle Analytics
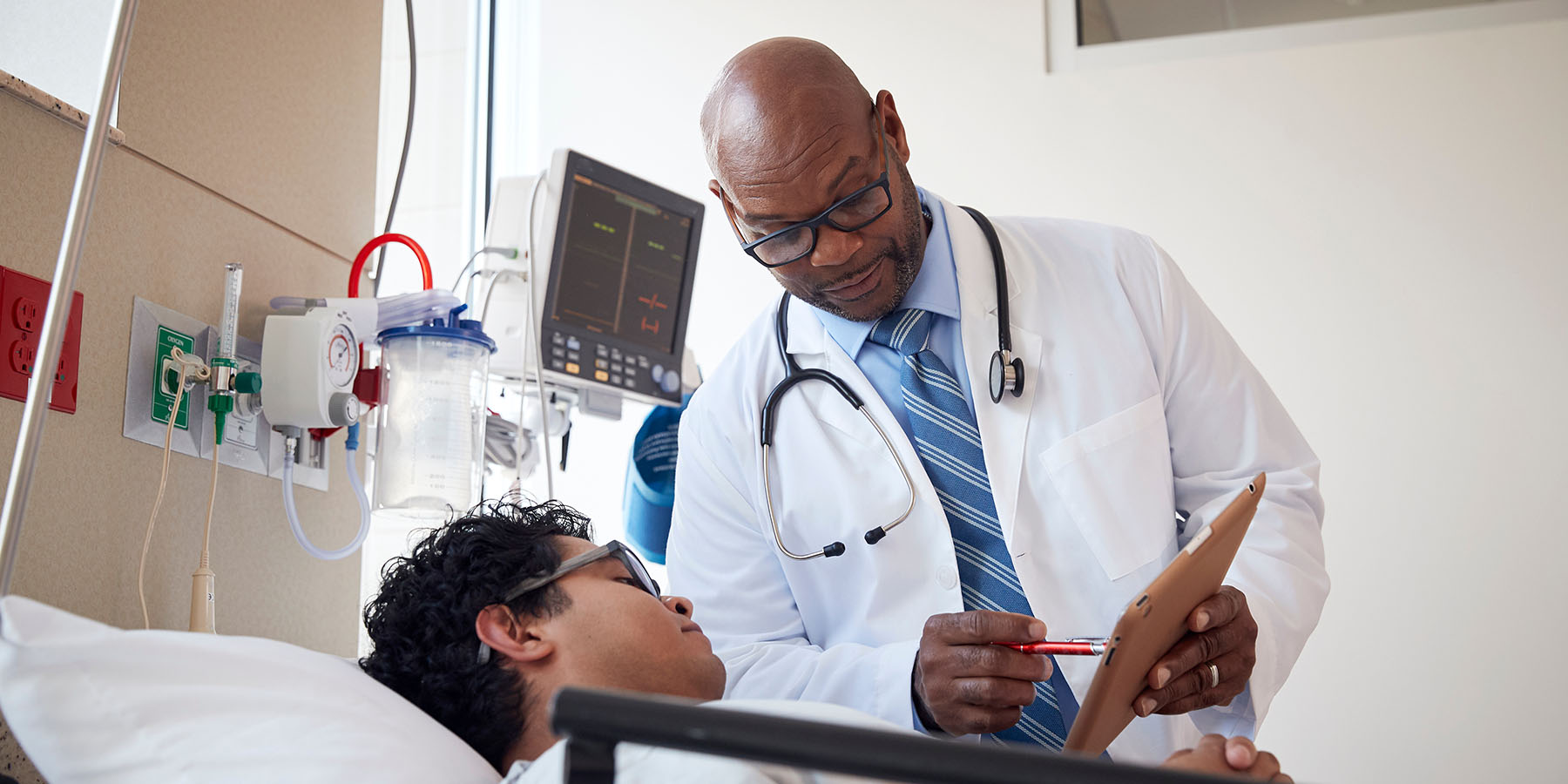
Data harnessed correctly will improve revenue cycle management. New technologies are key. A robust analytics strategy such as revenue cycle analytics can maximize effectiveness and reduce claim denials.
However, the benefits of integrating these tools extend beyond mere cost reduction and efficiency enhancement. More significantly, it opens up avenues to increased revenue generation and fosters financial stability.
Importantly, it's crucial not to overlook another profound benefit of employing revenue cycle analytics – the potential for enhanced patient care. Ensuring the provision of services is seamless, we can effectively eliminate needless delays. This ensures patients receive their much-needed care in a timely manner. By improving processes, we are, in effect, improving patients' experiences and outcomes.
In the infancy of AI and machine learning, the time for action is now. Healthcare providers should seriously consider investing in innovative technologies, especially ones such as revenue cycle analytics. These aren't just tools; they're catalysts for transformation.
They have the power to improve workflows, reduce mistakes, and bring about meaningful change. Technology will fundamentally change how we view and manage our revenue cycle.
It's not just about keeping pace with the ever-evolving world of healthcare technology. It's about actively shaping the future through the thoughtful adoption of innovation. By integrating revenue cycle analytics into our toolkit, we can not only react to changes but anticipate them. This proactive approach ensures that we stay ahead, driving improvements in our processes, patient outcomes, and bottom lines.
The future of healthcare is data-driven, and revenue cycle analytics stands at the forefront. We can use the power of healthcare providers to create a financially secure future. This will also lead to a healthcare ecosystem that is more focused on the needs of patients.
A Quick Win: Gistia RevenueIQ
Gistia RevenueIQ is a low-risk, quick-win solution. It uses revenue cycle analytics to improve revenue cycle management.
Gistia RevenueIQ helps you prepare for future trends, prevent claim denials, and make workflows faster. This will benefit your organization and, most importantly, the patients you serve. It will create a brighter and more sustainable future.
Frequently Asked Questions
How can data help prevent claim denials in healthcare organizations?
Data can help us identify patterns in denials. This allows us to uncover their root causes. We can then develop strategies to prevent them from happening again. By recognizing these patterns, providers can make necessary adjustments to their internal processes.
What is predictive revenue cycle analytics?
Predictive revenue cycle analytics is a method that uses historical data. By applying advanced algorithms it can identify denied claims.
Providers can anticipate potential issues before submitting their claims. This foresight allows them to take a proactive approach to revenue cycle management. Correcting issues beforehand prevents problems down the line.
How can metadata be useful in revenue cycle analytics?
Metadata, or information about the data, can provide invaluable insights to augment operations. Predictive revenue cycle analytics can help improve data-driven decision-making. It does this by focusing on the quality of data in the context of the entire revenue cycle. This provides crucial insights into potential problem areas.
What are the benefits of using revenue cycle analytics in healthcare organizations?
Benefits include the ability to identify problem areas, understand them, and take preventive measures to curb their recurrence. This strategic and ongoing method optimizes processes, reduces denials, refines revenue cycle management, and ultimately leads to improved patient outcomes.
How can revenue cycle analytics improve patient care?
Processes improve - eliminating needless delays and ensuring patients receive care quickly. This contributes to improved patients' experiences and outcomes.